Do you realize that your business starts acquiring data on Day 1 of its existence? All it takes is a website and electronic payments, and voila, you have data! Once you have a social media presence, more data. You make some marketing efforts, start capturing leads, the data just keeps flowing in.
For a brief while, you may be simply sitting on this treasure trove of data. But very soon, you’ll need to get up and build a team that makes all this data work for you. In this primer, we give you the step-by-step details on how a fast-evolving startup or SME can build a data team from the ground up.
When do you need a data team?
Ideally, your business should look to build its data team when it starts selling and has a semblance of repeatability in customer acquisition. At this point, the data team can start adding a lot of value. Here are some examples:
- Customer segmentation: understanding different target audience or groups, and tailoring the sales approach to each
- Lead scoring: identifying the leads most likely to convert so sales efforts can be focused
- Sales forecasting: looking at various data sources such as CRM, marketing campaigns, seasonality, industry trends, etc. to forecast sales.
Thumb rule: You should definitely build a data team before hiring a VP of Sales; they can run operations much faster with the services of a data team.
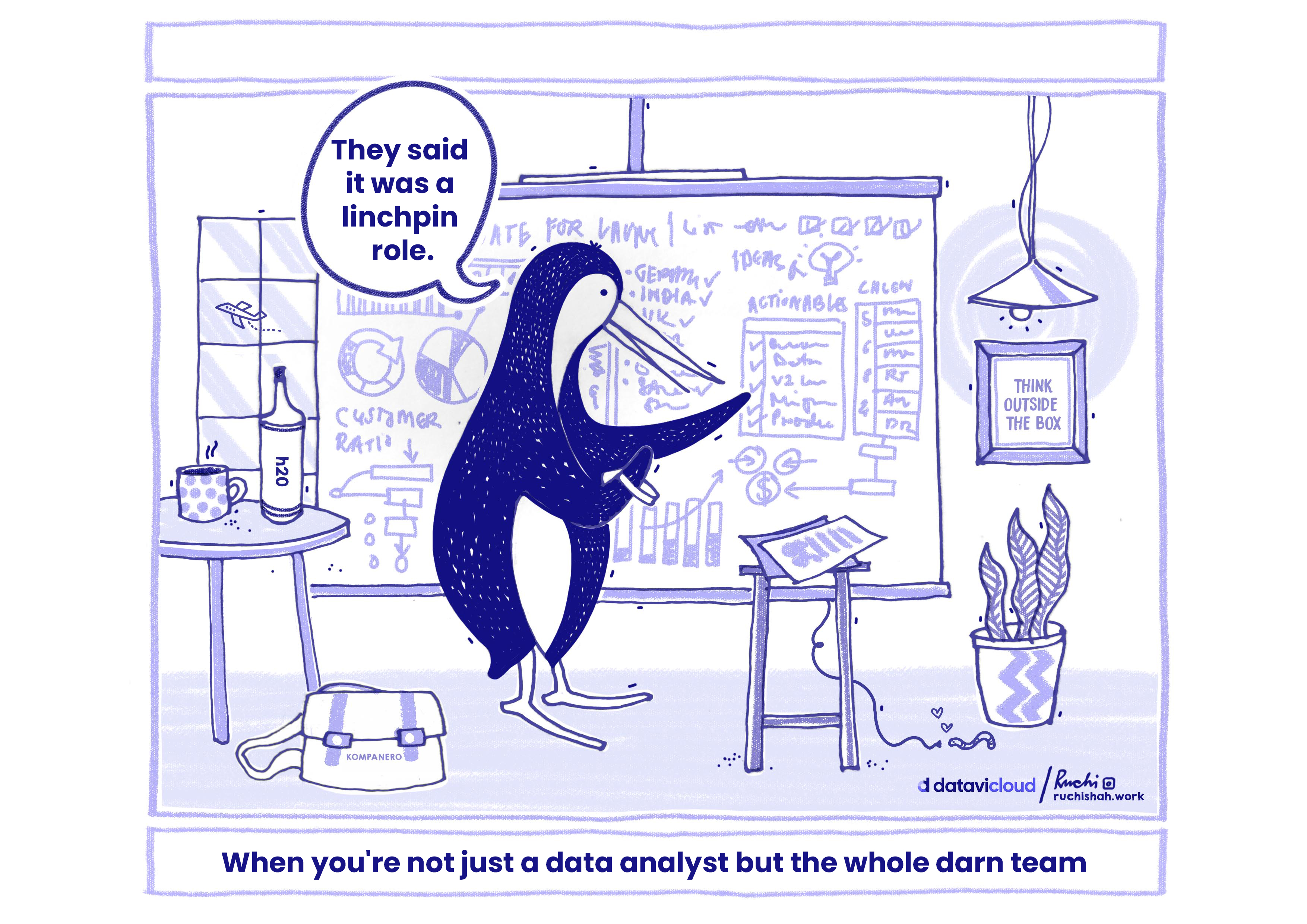
Building a data team: where to start?
As all great thinkers say, start with the end in mind. i.e. set the objectives you want your data team to achieve. Rope in key stakeholders from different teams - engineering, product, marketing etc, to formulate these objectives - because many of them will ultimately be the collaborators and internal customers for your data team.
If your business is in its nascent stages, your data environment probably is too. The type of analytics you will deal in is likely to be descriptive. That is, simply looking at what has happened so far by looking at your data sources. Over time, the objectives of your data team may shift to diagnostic analytics - that is, why did something happen?
As your data environment matures, and you dig even deeper into your data, you will likely move towards predictive and prescriptive analytics (what will happen/how do we work towards an outcome?) We have covered this in more detail here.
Think long and hard about what your organization needs from your data team in the short to medium term, so you can hire accordingly.
Who should your first hire be?
The best data teams start with one great data hire, typically a generalist who can do a bit of everything and scale the team up when necessary.
When we say a little bit of everything, we’re looking for somebody who can:
- Develop insights from data. For instance, analyzing customer purchase patterns to identify the most popular products and key demographics.
- Develop business hypotheses. Like using these insights to formulate product bundles targeted at certain demographics.
- Create a scalable data architecture from scratch - creating cloud-based solutions and ETL pipelines to collect, process, and store all this data your startup is collecting from various sources - customer transactions, website analytics, campaigns etc.
Our take: Hire a generalist with strong business acumen, SQL capabilities, and 3-5 years of experience building or working with a data team at a startup. Somebody who is able to eat, breathe, and live data, and can find and solve business problems.
Responsibilities of an early data team
The early data responsibilities will include the following, scaling up as the business evolves:
- Translating business requirements into a strong data and analytics setup.
- Extracting insights from large, complex data sets using strong database skills.
- Improving business processes and influencing how other business functions work using data-backed insights.
- Communicating findings to stakeholders and taking initiatives to improve business performance.
- Presenting data and analyses in a format appropriate for a varied audience using data visualization, dashboards, or other methods.
- Automating and building lasting solutions that frees up their bandwidth to work on higher level problems.
What we are building at DataviCloud is designed to help data teams do all of these better and faster. The product will be open to early users soon - join the waitlist if you’re curious.
How big should your data team be?
Data teams don't really need to scale linearly as your organization grows. So long as there is a focus on automation, efficient triaging of ad hoc requests (i.e. the data team can quickly and effectively assess the urgency and importance of these requests and figure out an order of priority) and proactiveness in addressing business requirements, a team of 2-3 can support the decision making for an organization with up to 100 employees.
Once your business grows to say, 1000 employees, your data team should also have grown to 12-15 to handle complex analytics requirements. You will need a well-oiled data team, with a mix of data engineers, data analysts, and data scientists.
But wait, what’s the difference between the three?
In many young organizations, the boundary between these roles is fluid. You might have an all-in-one data handyman (architect, analyst, scientist, rolled into one). This happens all too often, as Reddit will tell you.

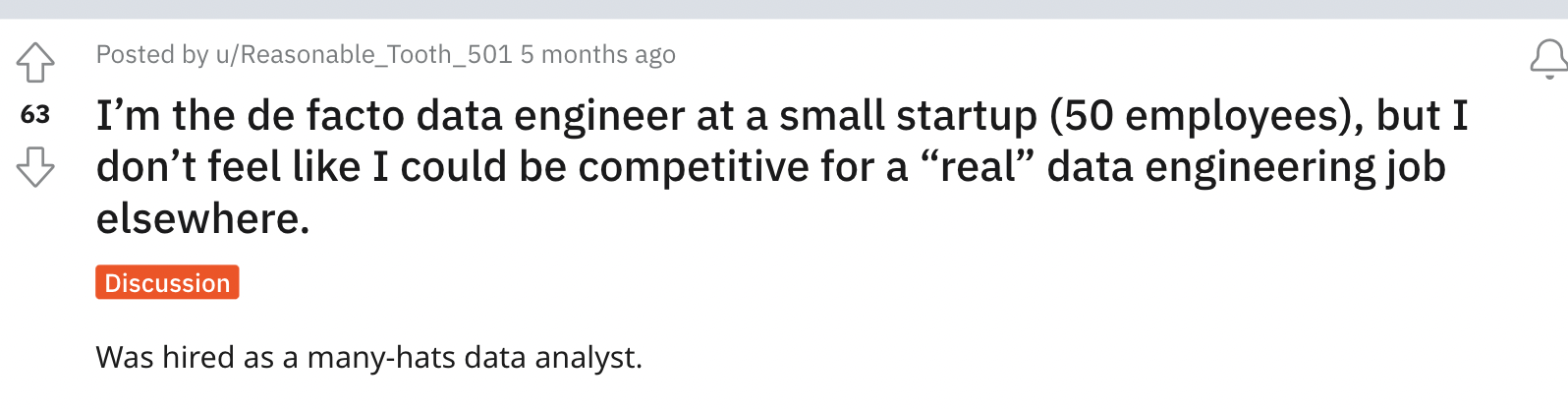
But there are well-defined differences between the three roles and they will become significant as your team grows. We’ve summarized them here.
What is a data analyst?
A person who gathers, reviews, and analyzes data to identify data-led insights which inform critical business decisions.
Data Analyst skills include:
- Data visualization
- Data warehousing
- Stakeholder management
- Critical thinking
What is a data engineer?
A person who builds and optimizes the systems that allow data scientists and analysts to do their work.
Data Engineer skills include:
- Programming, handling Big Data.
- Knowledge of data ingestion, discovery and automation.
What is a data scientist?
A person who utilizes statistical analysis, programming, and domain knowledge to extract insights and solve complex problems from large datasets.
Data Scientist skills include:
- Machine learning
- Programming
- Statistical modeling
- Mathematics
When evaluating candidates, don’t get too caught up in their familiarity with the tools or platforms you use. In a nurturing environment, people can easily skill up at using new tools, if they have used similar ones before.
Here’s what to look for instead:
- Business acumen: Problem identification and articulation.
- Data science and visualization: Ability to find answers using data visualization and/or statistics/AI/ML models and sharing insights.
- Data engineering: Ability to ingest data into a platform, stitch it together, and automate.
It is difficult to find people with depth in all the three categories. And honestly, it's a little unfair to expect it too. Even if candidates are strong in two of the three areas, they can be rockstars at solving your business problems.
Where should you look for top data talent?
As you figure out how to recruit data analysts, engineers, or scientists, here’s something you should know. The traditional route of focusing your HR team on job portals still works. But, you’ll need to spend a lot of time filtering profiles to find candidates that fit your needs.
What might short-circuit your search is to look at referrals and analytics-focused groups on LinkedIn, Reddit, Discord groups, etc. Looking towards industry-agnostic analytics firms is a good bet too.
Analytics is one of the ‘hot’ careers of the day, and competition for top talent is intense. There are many firms with deep pockets matching and raising numbers, which make salaries unviable for businesses looking to keep operations sustainable.
How to attract great talent?
So what can you do to attract talent when you’re not the best paymaster out there? More often than not, offering fair monetary compensation with other benefits helps: a well-defined role with exciting challenges, access to leadership and decision making, and an environment that lets them pick up and apply new skills.
We also have a bias for choosing talent with potential and having a well-structured onboarding and training environment to nurture them, rather than throwing money at candidates with more experience. If you can afford it and they can really add value, go for it by all means. But don’t get dazzled by fancy resumes, especially if you aren’t there yet.
What does the hiring process look like?
Once applications start to come in and you’ve filtered a handful of profiles that show promise, here’s how to proceed.
Step 1: Fit Assessment
Hiring is a two-way engagement, with company and candidate both evaluating each other. Since job options are plenty, pitching the role well is crucial. Unless candidates are excited about the opportunity, they will not be able to give their best to the hiring process.
And if they’re excited, be willing to change your mind about your parameters. Vikas recalls a time when he did, “We met a candidate with exceptional raw talent but who did not have enough years of experience. But we took a bet on her, nurtured her, and provided challenges to help her scale up. She did amazingly and went on to lead analytics for a sales team later.”
Step 2: Technical interviews
Test relevant skills by going through the candidate’s previous projects in depth. Probe into problem areas - critical hurdles,sufficiency/inadequacy of data available in solving the problem, etc, with the help of whiteboarding. These will give you a good idea of their abilities. If you want to confirm some of your inferences, give them an assignment with a short turnaround time and evaluate their approach.
Step 3: Team and culture fit
Will the new member make your team stronger or more versatile in tackling analytics challenges? To answer this question, involve your team leads/members in the interview and talk to the candidate about their past experiences in teams, values, personal aspirations, and way of working.
Ask the candidate how they prefer to receive feedback and how they provide feedback to others. Assess their openness to constructive criticism and their ability to contribute to a positive feedback culture. You can also present hypothetical scenarios to evaluate their attitude to collaboration and conflict resolution.
Step 4: HR round
The primary purpose here is to cross-validate Step-3, and provide an independent take. Have a strong working relationship with your HR business partners, because their reading of the candidate will complement yours and help uncover any biases/blind spots.
Says Vikas, “I trust my HR BP partners even when they are going against my reading of the situation. There was a case when I was convinced about the candidate, but the HR changed my decision because they felt there was a mismatch between team needs and the candidate’s career aspirations. That person quit to pursue higher studies within 6 months of joining another firm.”
Technical skills to look for
A graduate degree or equivalent is a must-have. A background in statistics, operational research, or mathematics would be a definite plus. If you’re hiring before your learning and training setup is mature, look for candidates with at least a couple of years of work experience in Business Intelligence/Data Analytics/Modelling, preferably within an IT product, e-commerce, or SaaS company.
When it comes to core skills, most important is the ability to manipulate large data sets with high dimensionality and complexity; proficiency in SQL, Excel/Google Sheets, BI reporting tools (e.g. PowerBI, Tableau, Qlik, Looker Studio). It's a bonus if the candidate is proficient in a coding language such as Python or R.
Soft skills to look for
Data is a team sport and the data practice is by definition cross-functional - so soft skills and EQ are just as important as technical skills. The willingness to help each other and the courage to seek help, will go a long way towards fostering an effective team. Broadly, here are the soft skills to look for:
- Communication skills: Look for clear communicators who can adapt to technical as well as non-technical audiences.
- Ethics and security-first mindset: When you’re starting out, your processes are likely still vulnerable to privacy and security concerns. Hire people who have a no-tolerance approach to security and will lay the proper groundwork for data security.
- Flexibility and adaptability: Your data team needs ninja-level adaptability skills. Not only do they need to adapt continuously to the company’s growth and track it, they also need to keep their eyes and ears open to developments in the fast-changing data infrastructure and tools industry.
Setting up your data team for success
Hiring is just the first step of building an impactful data team. Onboarding is as important. Having a structured process to onboard and augment skill sets with training will keep your team nimble and up-to-date.
Next comes the business of nurturing your talent. What will keep your data team satisfied at your organization? While people have different outlooks and needs, there is a data analyst ‘type’, and here is broadly what he or she may look for: autonomy, impactful roles with clarity about roles, a clear data engineer roadmap/data analyst roadmap, proximity to leadership and the decision making process, and psychological safety that enables 'learning by doing'.
Another way of looking out for your freshly-minted data team is making sure that leadership has their back. Data teams often need executive sponsorship to clear the path for them, especially at the start, before they gain trust from business functions. They need access to meetings, and live or near real time data to be more impactful. If they are assured of these enabling factors, they can function smoother.
We’ve found that building a strong data team is one of the best investments we can make in our business - much as it may be challenging at the beginning. If you have best practices and learnings to share, we’d love to hear them!