When you’re in the thick of scaling a SaaS company, it feels like you’re juggling a dozen things at once—customer retention, product improvements, and finding that elusive product-market fit, and so on. But there’s a powerful tool that can help you make sense of it all. Cohort analysis.
Let me explain with an example from my time leading Data & Analytics at Freshworks. As with any SaaS product that works on a freemium model, one of the problems we were trying to crack was how to convert free users into paying users. We turned to cohort analysis to uncover the behaviors that differentiated converters from non-converters.
The results were eye-opening: users who engaged with certain features within the first week of signing up were significantly more likely to convert to a paid plan. Armed with this insight, we doubled down on making this feature more visible during onboarding. We saw a substantial boost in conversion rates soon after.
What Exactly is Cohort Analysis?
In the SaaS world, you're constantly collecting data on user behavior—how customers interact with your product, when they sign up, how often they use certain features, and so on. However, when you look at this data in aggregate (i.e., as a whole), you might miss important nuances. Cohort analysis allows you to break down this data into smaller, more meaningful groups (cohorts) based on shared characteristics or behaviors. Tracking these cohorts over time can reveal patterns and trends that are often invisible in traditional data analysis.
Example 1: Understanding Customer Retention
You notice that, overall, your customer retention rate is 60% after three months. While this aggregate data is useful, it doesn’t tell you much about why some customers stick around while others churn.
With cohort analysis, you could break down your users into cohorts based on the month they signed up. By analyzing these cohorts separately, you might discover that customers who signed up in January have a retention rate of 70%, while those who signed up in March have a retention rate of only 50%. This granular view helps you pinpoint when and why retention rates vary.
- Why: The January cohort might have benefited from a more successful onboarding process or feature launch, explaining their higher retention.
- How: You could investigate how the onboarding process or product features differed between these months.
- When: Identifying the time period when retention began to drop (e.g., after a specific product update) can help you address issues early on.
Example 2: Improving Customer Lifetime Value (CLTV)
Let’s say your SaaS company offers a subscription-based service with multiple pricing tiers. Your goal is to increase CLTV, but you need to understand what drives customers to upgrade to higher tiers.
By creating cohorts based on the tier customers initially sign up for, you can analyze their subsequent behavior over time. For instance, you might find that users who started with the basic tier in Q1 are upgrading to premium at a higher rate than those who started in Q3.
- Why: The Q1 cohort may have been part of a successful targeted marketing campaign that convincingly communicated the benefits of upgrading.
- How: Understanding how this cohort interacts with your product—such as which features they use most—can provide insights into what drives upgrades.
- When: By identifying the time it takes for users to upgrade (e.g., within the first two months), you can tailor your marketing efforts to encourage similar behaviors in future cohorts.
Thus, cohort analysis is like using a magnifying glass on your data, allowing you to focus on specific details and uncover patterns that are otherwise hidden in the big picture.
Cohort Analysis Use Cases for SaaS
The granular insights revealed through cohort analysis help you to take specific, data-driven actions, across functions. Here are some examples.
- Onboarding Effectiveness: Your onboarding process is crucial for setting the stage for long-term user engagement. Cohort analysis helps you see how tweaks in the onboarding flow impact different user segments. If you notice that certain cohorts have higher retention rates, you can replicate the onboarding processes that worked well for those groups.
- Targeted Marketing: Understanding which cohorts are more likely to upgrade can inform your marketing strategies, helping you focus on the customers most likely to increase their CLTV.
- Feature Adoption: Is that new feature improving daily active users or feature-specific usage rates? By tracking how different cohorts of users engage with the feature, you can quickly gauge its success and iterate if needed.
- Product Development: Cohort analysis can reveal critical moments when customers either drop off or decide to upgrade. These insights are gold when it comes to product development. If you see a trend where users are abandoning ship after interacting with a particular feature, it’s time to reassess and improve that area. Conversely, if a feature is driving upgrades, you’ll know it’s worth doubling down on.
- Pricing Experiments: Pricing changes can make or break your revenue streams. By segmenting users based on the pricing plan they were offered, you can see how these changes affect conversions and churn.
Even better, the results from a cohort analysis can be correlated to other key metrics to discover more. For instance, you’ve run a marketing campaign and want to see if the new users it brought in are sticking around and contributing to your revenue. By correlating their cohort data with MRR, you can quickly assess the long-term impact of your campaign and fine-tune your strategy accordingly.
The Pitfalls in Cohort Analysis
Missteps in how you set up and interpret your cohorts can lead to misleading insights or, worse, poor business decisions. Here are a few that I’ve come across most often.
- Not Keeping Data Snapshots
Because user behavior and interactions can change over time, it’s important to keep periodic snapshots of data. Without these snapshots, your analysis might be based on incomplete or outdated information, leading to inaccurate conclusions.
Say, your team is tracking user engagement over a 12-month period. In January, you introduce a new feature that you believe will improve retention. However, you don’t take a snapshot of user data before the feature launch. Six months later, you want to compare user engagement before and after the launch.
But without the pre-launch data snapshot, you can’t accurately assess the feature’s impact. Any conclusions you draw might be skewed by changes that occurred in the months following the launch—such as seasonal variations or other external factors—making it impossible to isolate the effect of the feature itself.
To avoid this, make sure you’re taking regular snapshots of your data—ideally monthly. This will let you track changes over time more accurately. This is where DataviCloud can massively simplify your life—with Automated Data Snapshots. DataviCloud automatically keeps monthly snapshots of your data, ensuring that your cohort analysis is always based on the most accurate and up-to-date information.
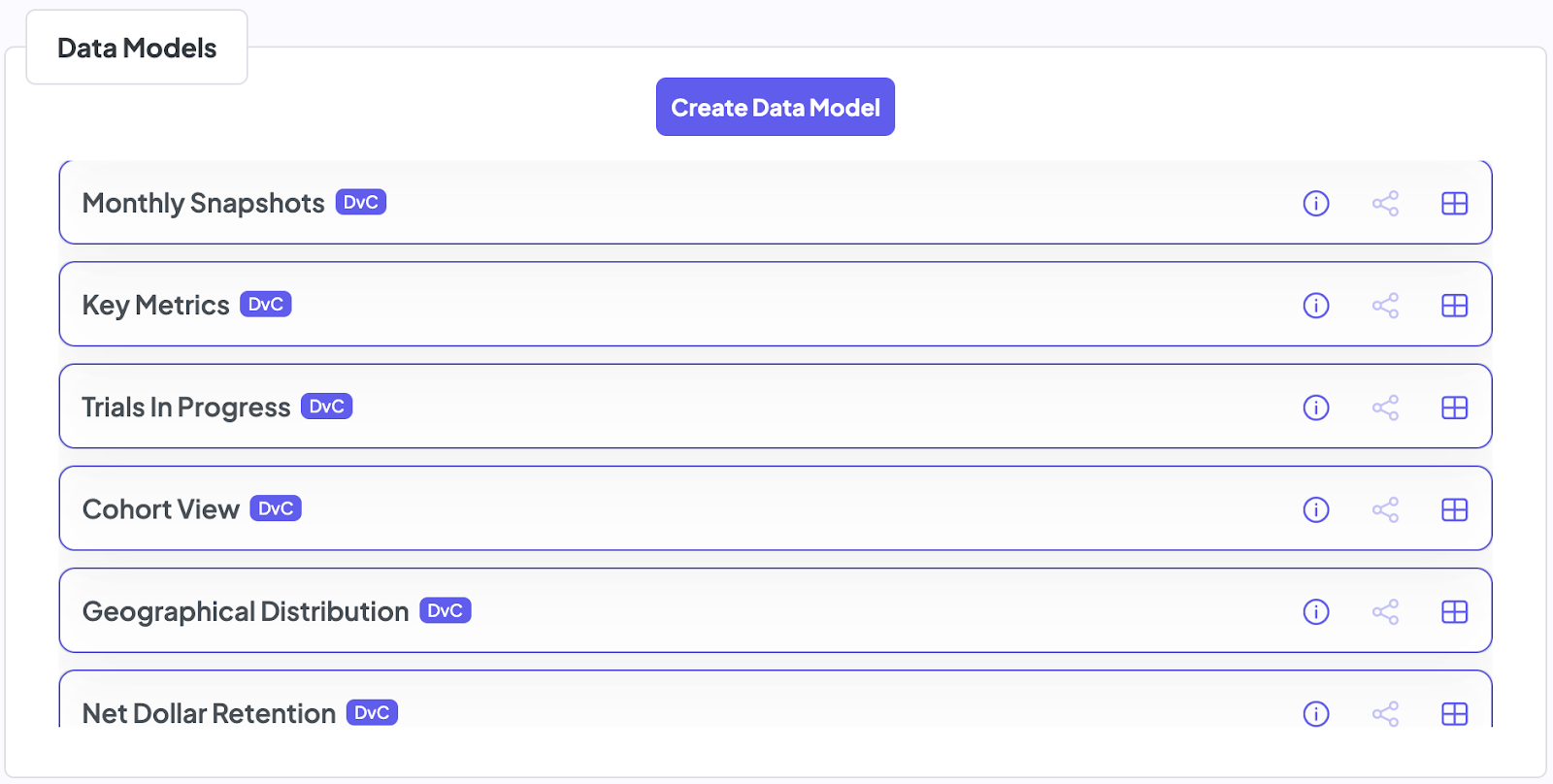
- Poor Segmentation
Another common pitfall is defining your cohorts too broadly or too narrowly. Poor segmentation can lead to cohorts that are either too general to provide useful insights or too specific to be actionable.
Say you’re analyzing customer churn for an email marketing platform. You decide to segment users based on the month they signed up. After running the analysis, you find that the churn rate is relatively consistent across all cohorts, leading you to conclude that churn isn’t a major issue.
However, this broad segmentation overlooks critical nuances. What if users who signed up through a specific marketing channel (like a webinar) are churning at a much higher rate than those who came through organic search? Without more granular segmentation—such as breaking down cohorts by both signup month and acquisition channel—you might miss these important details.
To avoid poor segmentation, start by clearly defining the specific questions you want your cohort analysis to answer. Then, segment your cohorts in a way that aligns with these questions. For instance, instead of just looking at signup month, consider factors like acquisition source, product usage patterns, or user demographics. DataviCloud has robust Segmentation and Backtesting capabilities that make it easy to define and refine your cohorts with zero coding or grappling with spreadsheets. This will help you uncover more meaningful insights that can drive actionable strategies.

- Misinterpreting Correlation as Causation
It’s easy to fall into the trap of assuming that because two trends occur together, one is causing the other. However, correlation doesn’t always mean causation, and this can lead to misguided decisions.
Suppose you notice that users who interact with a certain feature within the first week of signing up have a higher retention rate. You might conclude that this feature is driving retention, so you decide to promote it heavily during onboarding. But what if it’s not the feature itself that’s causing users to stick around, but rather the fact that highly engaged users—those who would likely stay anyway—are the ones most likely to explore new features early on?
In this case, you might pour resources into promoting a feature that isn’t the real driver of retention, missing the opportunity to focus on what truly matters. To avoid making this mistake, use cohort analysis in conjunction with other data analysis methods, such as A/B testing, to confirm your hypotheses. Look for additional evidence before making significant business decisions based on observed correlations.
------
Way back in 2012, Christoph Janz, early-stage SaaS VC and Managing Partner at Point Nine, wrote this: “If you're a young, bootstrapped startup and you have to decide if you want to use your developers' scarce time to improve your product or to get better statistics most founders will decide for the product. That's understandable. Nonetheless, I would like to argue for a high-quality standard of metrics early on, since the insights that you'll get by understanding your metrics will often be highly actionable. And of course, it will make your conversations with investors who want to understand your numbers much easier.”
Janz’s advice highlights a crucial point: while it’s tempting to focus solely on product development in the early stages, ensuring that you have a robust data collection and analysis framework in place is just as important. The insights you gain from understanding your metrics—especially through tools like cohort analysis—can guide your decisions and provide the clarity you need when speaking with investors or planning your next move.
If you’re not already leveraging cohort analysis, now is the time to start. And with DataviCloud, you can make the process not only easier but also more powerful.
Sign up for an outcomes-focused demo on www.datavicloud.com.