Here at DataviCloud, we love a good data analytics success story. The best ones stand out for their prescience, audacity of thinking, or just the consistency and thoroughness with which they leverage data to improve their operations, drive growth, or deliver a stellar experience to their users. We’ve rounded up a few of our favorites.
Tesco: The oldie, the goldie

Back in the 90s, long before the explosion of online shopping and relentless data collection, UK-based grocer Tesco launched a rewards scheme called Tesco Clubcard. Every time a customer made a transaction, they’d earn points on their Clubcard and Tesco would collect a record of the sale, associated with the customers’ name and address.
With this, Tesco figured out who their most loyal customers were - the ones who would drive long miles to come to their store, the ones that kept coming back, and the ones that accounted for a big percentage of their sales. This intel helped Tesco to create tailored offers for shoppers and reward high spenders.
By today’s standards, this exercise sounds very basic. But it was, in effect, an early inroad into data analytics for Tesco, one that helped it become the UK’s largest grocery store chain. It also ushered in the age of rewards schemes not just by retailers but across industries.
Target: Calling new moms and dads

Another decade, another retailer, another strategy. In the early 2000s, Target set its statistician, Andrew Pole, to work to figure out which of its customers were pregnant. For retailers, new parents are a lucrative demographic. With a baby on the way, to-be-parents buy (and sometimes, over-buy) supplies and form brand loyalties that last for years, if not decades. It’s a narrow, important window in time — and Target decided to go after it.
What Target came up with was a ‘pregnancy prediction’ score, arrived at with the help of various input factors. They noted that pregnant women often bought calcium and zinc supplements in the first twenty weeks, unscented lotion in the second trimester, and washcloths, sanitizers, cotton balls, etc. in the third trimester. By tracking which customers were buyers, Target tried to predict whether they were pregnant and when they were due, and began sending them custom coupons and using psychological marketing strategies to influence their spending.
If this level of insight into an aspect so personal unsettles you, you’re not alone. Target did get into trouble when it inadvertently outed a teenager’s pregnancy to her family. With Big Data comes big responsibility, which is why there should be watchdogs, both within businesses and outside of them, to ensure that ethical concerns are addressed.
Netflix: From mail-order DVDs to AI-crafted movie trailers
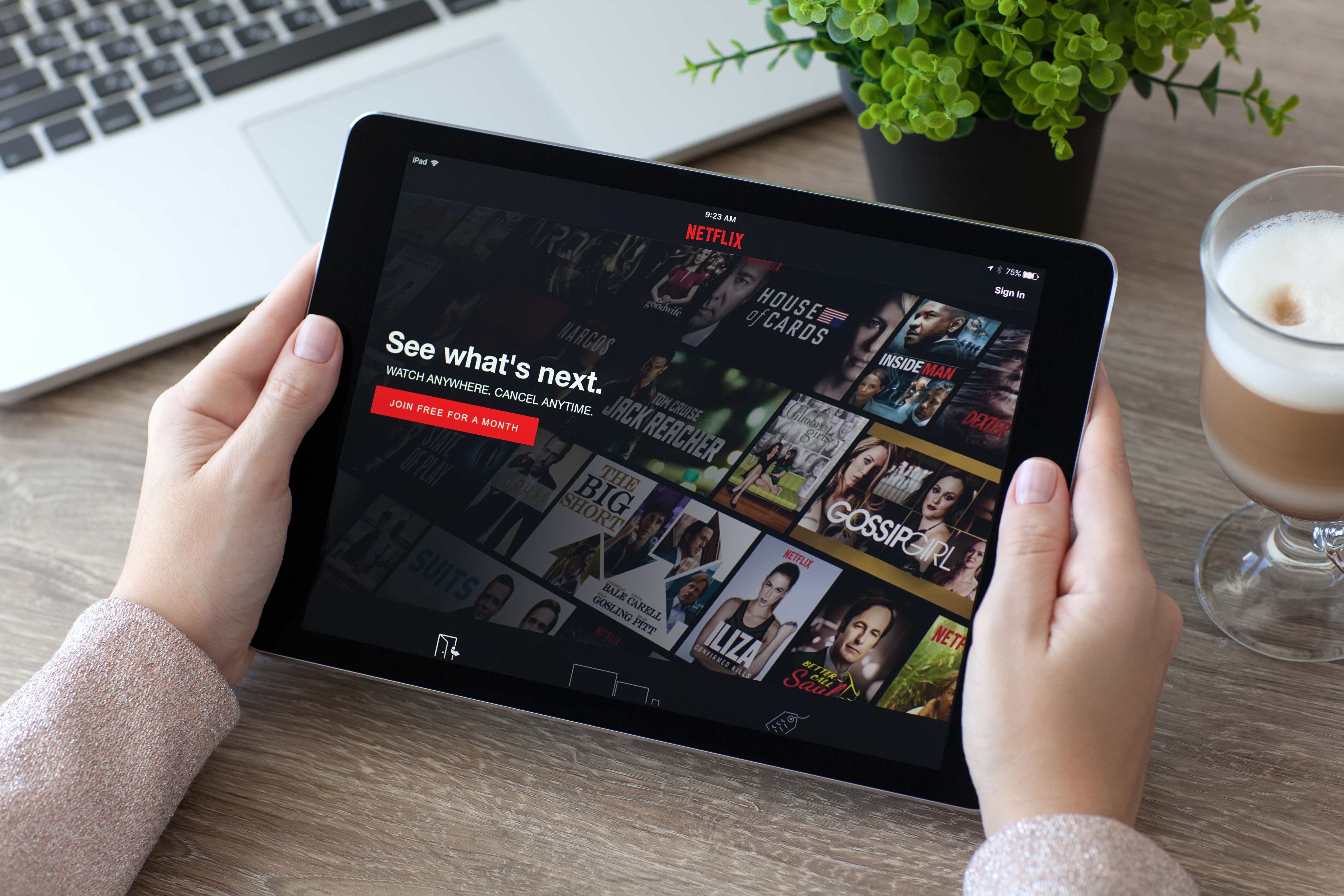
The poster child for digital transformation, Netflix’s every move is seemingly orchestrated by data analytics. Netflix has an astonishing 93% customer retention rate, far more than its competitors. What’s more, its foray into original content in the early 2010s began giving Hollywood a run for its money and awards haul.
All the data collected from its whopping database of 240+ million subscribers goes into Netflix’s recommendation algorithm. What a subscriber watches, how they watch it (Do they binge? Do they watch only over weekends?), when they lose interest - and pretty much every other detail of viewing behavior is an input to this algorithm.
In an earlier post, we wrote about building a data maturity model. As organizations mature, they typically move from descriptive analytics (answering the question ‘what happened?’) to diagnostic analytics (‘why did it happen?’) to predictive analytics (‘what will happen?’) and prescriptive analytics (‘how can we make it happen?’) Netflix has certainly followed this course.
They began by monitoring viewership data (descriptive), moved on to predicting what viewers might potentially like (predictive analytics), and used that intel to create programs like House of Cards and Arrested Development (prescriptive analytics). Enrique Dans, professor of innovation at IE Business School, writes, “The latest Netflix series is not being made because a producer had a divine inspiration or a moment of lucidity, but because a data model says it will work.”
Netflix has used AI to create customized trailers for each person. For instance, if a person likes comedy, they will be shown a trailer filled with comic scenes from the movie, even if the movie in itself is of a different genre, say drama/action.
Having already personalized artwork and trailers to show each person a version they are most likely to respond to, Netflix has recently begun creating personalized Dynamic Sizzles; as they write on their tech blog, “Now Netflix can create a personalized sizzle reel dynamically in real time and on demand. The order of the clips and included titles are personalized per member, giving each a unique and effective experience… we create Dynamic Sizzles with minimal human intervention.”
Asian Paints: The secret data company

Closer home in India is a possibly surprising contender for the best data-driven company—Asian paints, an 80-year old paint maker. Asian paints has always been something of a maverick. Way back in the 1960s, it disregarded the traditional distribution network of paint and operated with its own delivery network, thus saving up to 20% in margins.
It was also a tech visionary that bought its first mainframe computer in the 1970s, even before ISRO did! It has been using analytics since the 70s to forecast demand and bolster its supply chain, eons ahead of its competition. With its long history and reigning market share, it has a wealth of data about exactly what paint people buy at various times of the year, in different parts of India.
Leveraging this data, Asian Paints uses AI and ML predictive tools to forecast demand at a store level. Currently, they deliver paint directly to 70,000+ registered dealers nearly 3-4 times a day based on demand patterns. And their predictions are so on-point that 90% of the time, the delivered paint gets sold within 3 hours. Net result: while competitors spend 30-40% of the retail price on distribution, Asian Paints spends a mere 3%!
Myntra: Tailoring customer experience with analytics
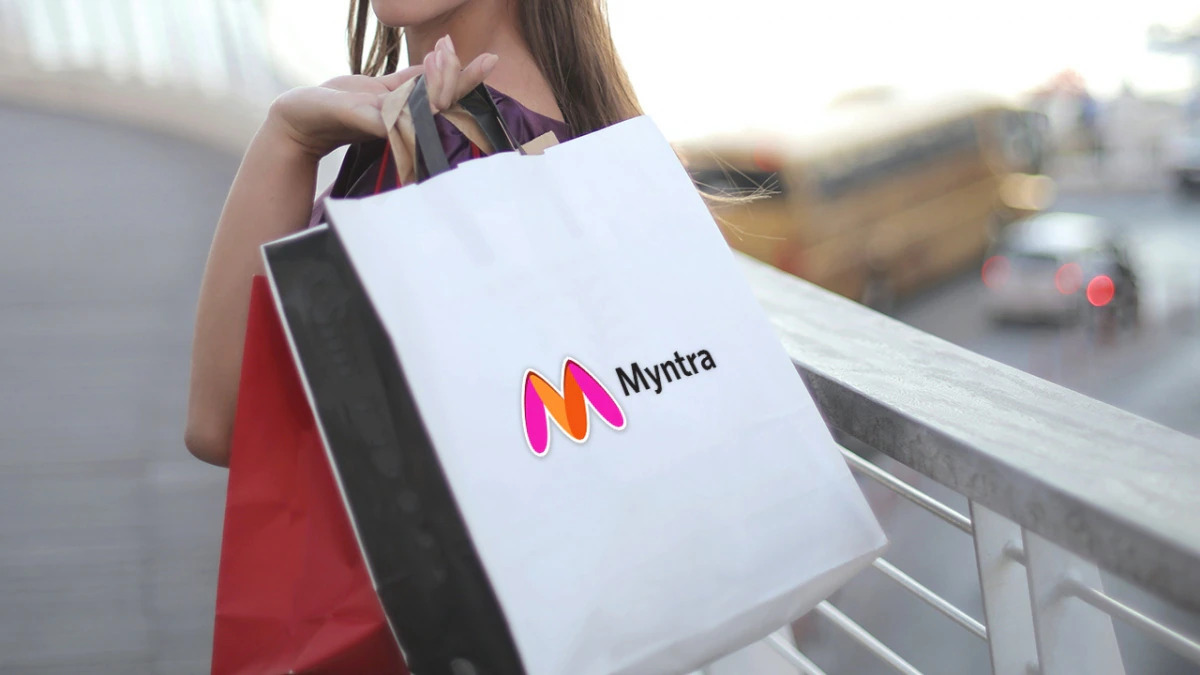
Myntra, a leader in India’s online fashion landscape, has been very vocal about how they are using data analytics and AI to make shopping more engaging and easier for customers.
By analyzing in-session browsing behaviors and information shared via customer profiles, they are able to offer personalized product suggestions that resonate with each customer's unique style. Even better, their ML models track user events within sessions and predict customer needs in real time. So if a customer who generally prefers western wear is browsing ethnic wear, the algorithm recognizes the temporary shift in intent and adjusts recommendations dynamically.
More recently, they have launched AI-enabled constructs such as My Fashion GPT and My Stylist to help customers put entire outfits together. My Stylist, for instance, lets you upload a photo of your own wardrobe or an image from the internet, combines it with information from your browsing and order history, and gives you super-personalized outfit recommendations.
Petrosea: Go data-driven or go home

Some years ago, Indonesian mining company Petrosea found itself at a crossroads. With increased regulatory requirements and pushback because of mining’s environmental impact, Petrosea had to make a hard shift or face extinction. So in 2018, they launched a company-wide effort towards sustainability through digitization.
At their Tabang project site, located in a remote corner of Indonesia, they deployed a new, comprehensive digital mining system to optimize fleet management - by reducing fuel consumption and truck allocations while taking into account road conditions in real time. This was achieved with the help of IoT - sensors attached to objects, advanced analytics, and machine learning.
The outcome of these efforts was remarkable: within six months, Tabang transformed into one of the company's most profitable operations, and the digital-first mindset soon spread through the entire organization.
Petrosea also deployed a suite of AI technologies to move away from coal and towards the mining of metals such as copper, nickel, gold, and lithium—the minerals required as electrification of developing countries. For these efforts and consequent results, Petrosea became the only mining company to join the Global Lighthouse Network, a community of manufacturers who’ve shown leadership in using Fourth Industrial Revolution technologies to transform business models.
The public sector: Data for better governance
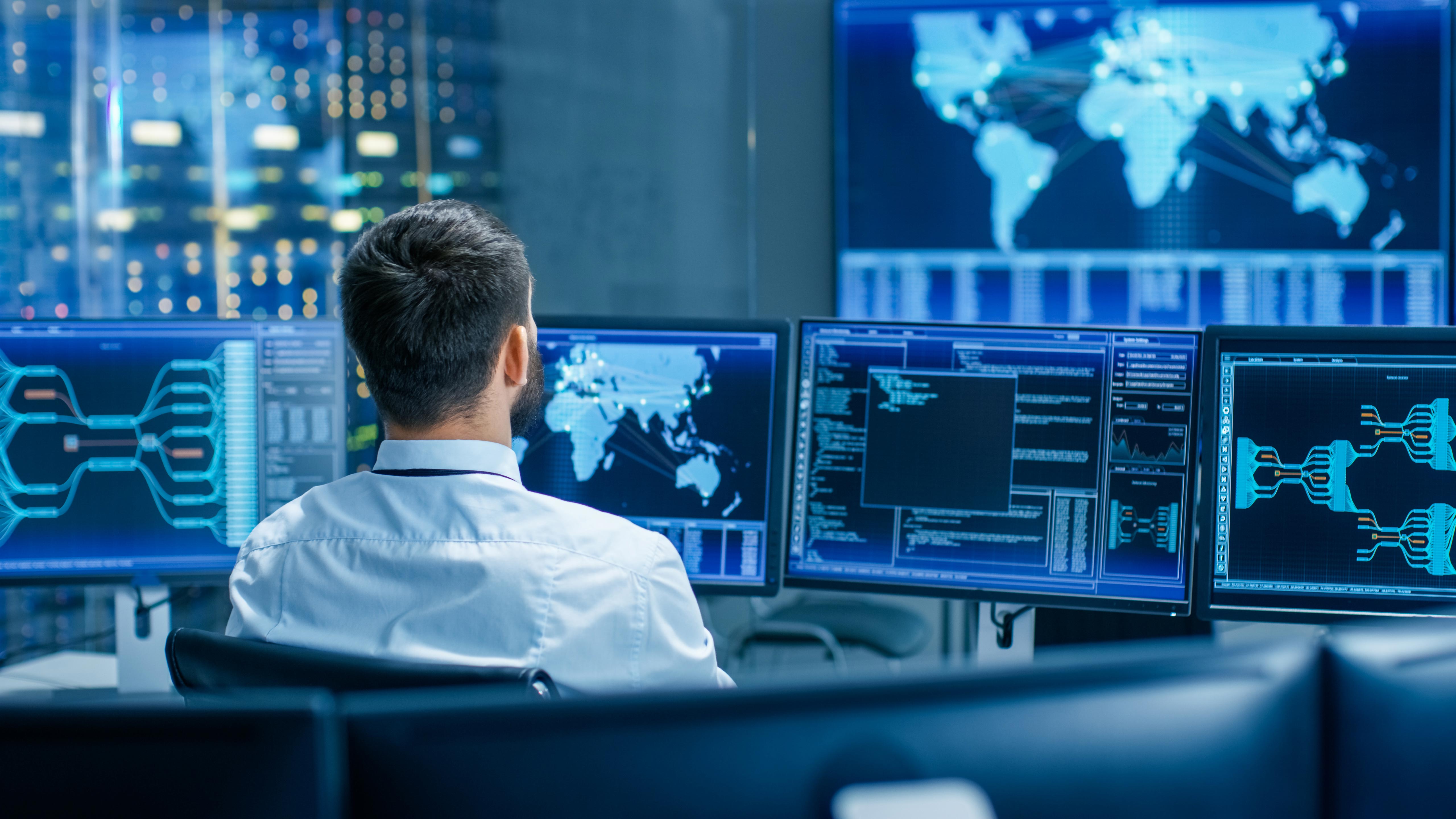
Governments and public sector organizations across the world are increasingly harnessing data to improve their decision making, detect fraud, monitor public health, assess economic trends, and so on.
In the US, the IRS formed the Office of Compliance Analytics in 2011, a team tasked with using advanced analytics to foster a data-driven culture. Within a few years, it delivered more than US$30b worth of improved tax compliance annually.
How does this work? A variety of methods are used to mine public and commercial data. This data is then combined with IRS’s proprietary information, and put through pattern-recognition algorithms that help identify refund fraud, detect taxpayer identity theft, etc.
Meanwhile, the stuff of science fiction movies is underway in Vancouver, British Columbia. Police are predicting break-ins with a machine learning solution that uses an algorithm to deconstruct crime patterns. Through spatial analytics, police can predict where residential burglaries will happen and plan patrols accordingly.
Here in India, data has brought new energy to the Indian Railways (IRCTC), which now employs AI and analytics for various aspects of day-to-day operations. IRCTC has introduced a predictive system which can estimate when maintenance is required by analyzing data from sensors installed on trains and tracks. Analytics is also being put to use to improve passenger experience, by looking at the data on seating preferences, seasonal trends, travel patterns, customer feedback etc.
Data is also serving a useful role in disaster management, among other things. In 2018, a prolonged spate of flooding in Bihar left more than 1400 dead. India’s Central Water Commission partnered with Google to create a flood-warning system that used AI, geospatial mapping and water data to generate flood alerts. The first alert was issued in September 2018, saving thousands of lives.
These are not all. From Zillow to Sephora, Tesla to Coca-Cola, there are hundreds of examples of organizations of all sizes across industries using data to their advantage and their customers' too. Do you have an interesting one to share?