Does your organization have a data glossary in place? If you don’t have one yet, please put it on your list of P0/P1s for this quarter and thank me later.
A data glossary is a crucial tool for data-driven decision making that is, unfortunately, often overlooked or relegated to the responsibility of the data team. But the fact is, a well-structured data glossary is even more important for business teams. Think of it as your organization's universal translator, turning jargon into plain business language so everyone from marketing to finance is on the same page.
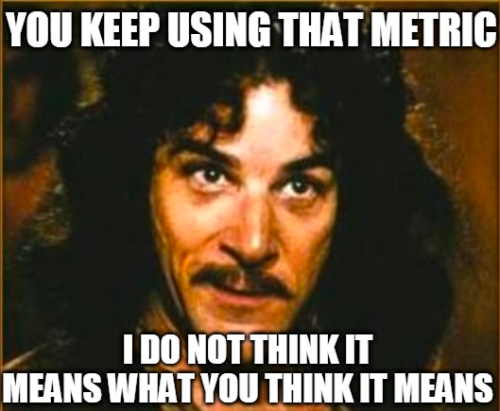
Let me give you an example. In a SaaS company, sales might define a "customer" as anyone who’s signed a contract or is actively using the product, regardless of payment status. They’re focused on closing deals, so once the contract is signed, that person is a "customer" in their eyes.
Finance, on the other hand, could see things differently. For them, a "customer" might only count once payment has been received. They care about recognized revenue, so if the customer signed up but hasn’t paid their first invoice, they’re still in the "pending" category.
This creates a situation where sales is celebrating new customer wins, but finance is saying, "Not so fast," because those wins haven’t hit the books yet. A data glossary bridges that gap, ensuring everyone knows what stage of "customerhood" they’re talking about.
A data glossary ensures that everyone is working with the same definitions, reducing confusion and helping teams collaborate more effectively. It doesn't just enhance understanding of commonly used (or misused) business definitions, it also helps to streamline workflows, improve productivity, and ensure data accuracy.
Data Glossary: Benefits for Different Users
1. Data Engineering Teams: Becoming Data Enablers, Not Gatekeepers
Imagine a day in the life of a data engineer. They’re bombarded with questions: "What does this field represent?" or "Where can I find this data?" Without a data glossary, they end up spending hours responding to these basic questions, instead of focusing on more strategic analysis.
Companies like Splunk have emphasized how a well-structured data glossary not only reduces the burden on engineering teams but also democratizes data access. When you have a central source of truth, teams downstream can answer their own data-related questions. This frees up data engineers to focus on critical tasks like optimizing infrastructure, rather than firefighting misunderstandings.

2. Analysts and Product Managers: Gaining Independence and Speed
In fast-paced environments, waiting on data teams to pull reports can be a major bottleneck. A well-structured data glossary turns analysts and product managers into self-sufficient problem solvers. This allows them to answer pressing business questions quickly and accurately.
Companies such as CoreBTS have highlighted how business analysts rely on data dictionaries to manage business intelligence requirements. When data is standardized and documented, analysts can easily query the data themselves, without having to consult the data team every step of the way. This speeds up the entire process and ensures consistency in the insights provided to leadership.
3. AI and Machine Learning Teams: Laying the Foundation for Innovation
AI and ML teams rely heavily on the structure and clarity of data. Without a comprehensive understanding of the data they’re working with, their models can produce inaccurate or skewed results. A strong data glossary becomes the backbone of creating robust algorithms.
Take a look at what NASA’s Planetary Data System (PDS) has done with its data dictionary, which provides exhaustive metadata for planetary objects. This enables scientists and machine learning teams to run accurate models and uncover hidden insights that would otherwise be missed. Having clear data definitions ensures that AI/ML teams can build futuristic tools, like conversational AI systems, without getting bogged down by inconsistent or incorrect data. This is why it is critical to have these created with automated systems and not have them generated manually.
4. Leadership: Trusting the Data Behind the Decisions
For leaders, accurate data is the foundation of informed decision-making. But if different teams are using different definitions of key metrics, or worse, pulling data from unreliable sources, the entire decision-making process becomes compromised.
At companies with the best data practices, the introduction of a business glossary and data dictionary helps standardize business metrics across departments. This creates a single source of truth, reducing discrepancies in reports and giving leadership the confidence that they were making decisions based on reliable data. Leaders no longer have to second-guess whether their teams are looking at the right numbers.
Why don’t more teams build Data Glossaries?
A general reaction when the data team hears about a data dictionary: Oh no! Why this overhead on top of all the ad hoc requests and repetitive tasks we have to do just to keep the lights on? This is the kind of seemingly boring and laborious stuff data analysts hate. More so in a lean set-up, when all this lies with a limited set of people, mostly in their brains.
That’s because building a data glossary is like planting trees. It's the right thing to do but it takes time and effort when done the manual way. And rarely will it be you (the OG team building it) who will benefit from the hard work.
Simplifying Data Glossaries with DataviCloud
As a team with data in our DNA, we want to take the pain out of creating a data glossary. Which is why we’re developing a plug & play Glossary Builder that automates it for you while also letting you customize and update it based on your needs. These are our building principles for this feature.
- Remove blank page syndrome.
- We are developing a pre-built data dictionary, business glossary, and data catalog so that data teams don't have to start from scratch. This has been our consistent approach across DataviCloud and makes as much sense here.
- Analysts can add to the already available base of business definitions, purpose, and typical use cases for each table/field.
- We will also provide sample queries and a collaboration/feedback mechanism to comment on or rate datasets, share tips, and contribute to ongoing documentation efforts to improve the catalog.
- Feature will be baked in, not bolted on.
- Our data glossary will use tags already present in the product like "Sales", "Finance", etc. to help users find assets easily.
- It will feature the name of creators/enablers against assets as data owners and stewards to improve data tracking and ownership.
- It will also include pre-built data quality metrics such as completeness, freshness and accuracy.
- Aiding productivity, less busywork.
- Our data glossary will highlight frequently used tables and have an easy Search functionality so you can find datasets using keywords, metadata tags or filters.
- We will have a conversational interface to simplify getting help with definitions, queries, etc.
- In future versions, we want to integrate AI to parse through the queries and table schemas to figure out primary, secondary and composite keys.
With DataviCloud, you'll have a ready-to-go, customizable glossary that grows with your organization, simplifying your data management and boosting clarity and productivity across teams. Book a call with us today for a free audit of your current data assets to know more.