You know how after you shift homes, you unpack almost everything, but stuff some boxes in the attic out of exhaustion - where they collect dust until your next move?
For an organization, dark data is those forgotten boxes in the attic.
It is data that is unknown, unused, and untapped - data that has fallen through the cracks of your organization’s machinery. Think of the troves of log files, customer chats, old campaign data, or email history gathering virtual dust in data repositories. These could be goldmines of insights for predictive analytics, risk management, and customer experience enhancement.
In today’s post, we confront the complexities of dark data – its potential to drive innovation, the inherent risks in its neglect, and how we can gain strategic insights by illuminating it.
How much data are we talking about?
Dark data is often colossal in volume. So much so that Gartner equates it to dark matter in physics. According to Seagate’s Rethink Data Report, only 32% of data in enterprises is put to use, while 68% goes unused.
Dark data floods in from every corner of your business. From the countless daily interactions between humans and systems, from server log files, IoT devices, sensors, unstructured text on social media, and much else. Unless you are constantly on top of your data game, dark data can very quickly get out of hand - and it often does.
Why does data remain in the dark?
In an ideal setting, all organizational data would be accounted for, connected, and put to work. In practice, much of it slips between the cracks. Here are a few reasons why, some systemic and some human-influenced.
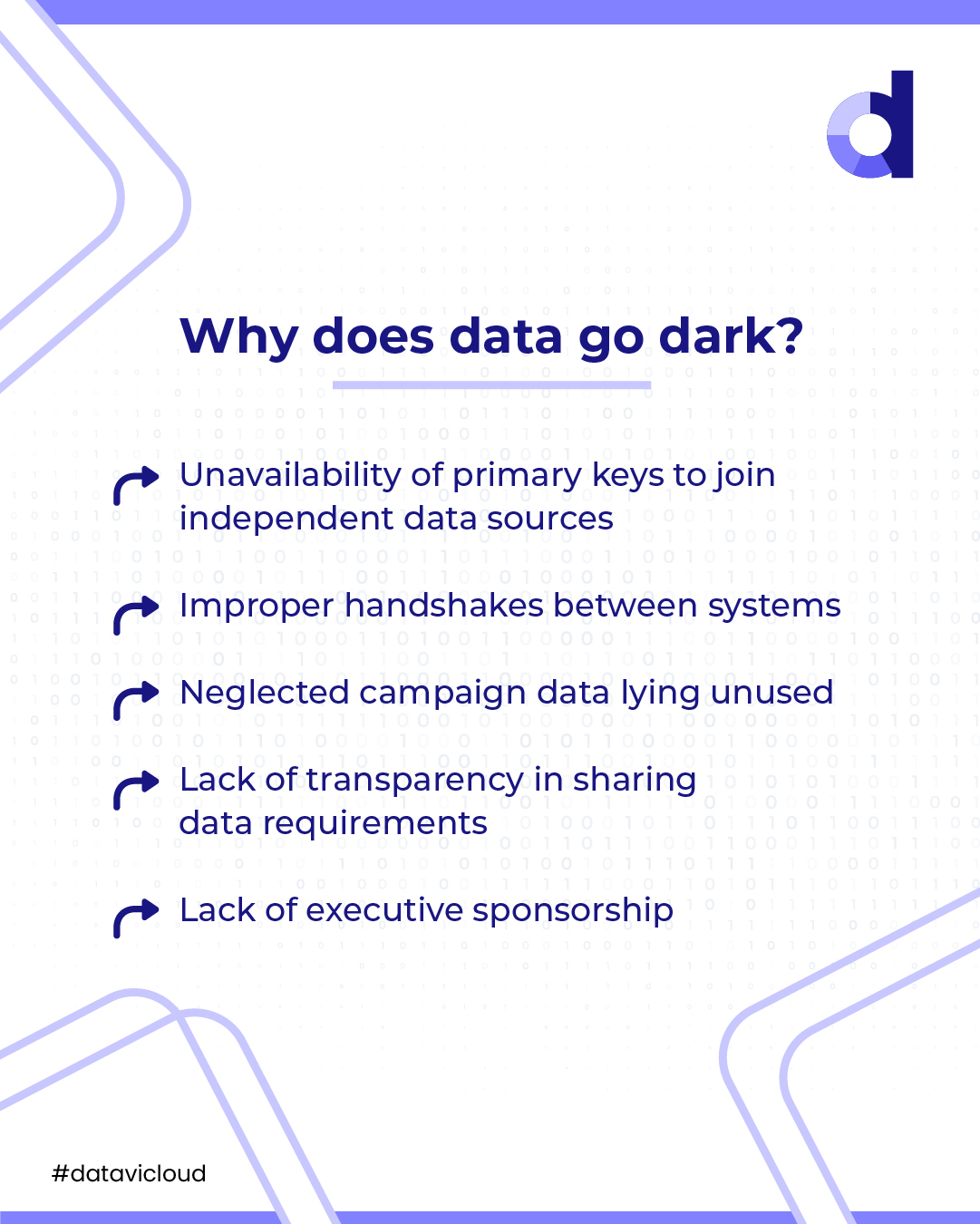
1. Unavailability of primary keys to join independent data sources
A primary key is a unique identifier that’s distinctive for each record, which helps you connect different data sources or silos. When there’s no clear primary key, you end up with different data sets in isolation. This can happen when the heading of column names for the same information is different across different data sources. Or sometimes, when the fill rate of the primary key field is low, and thereby has missing data issues.
2. Improper handshakes between systems
Even when two data systems are connected, an improper handshake between them could lead to data gaps. Consider a scenario where the sales department and the marketing department use different CRMs, for historical reasons. Normally, these systems interact seamlessly.
However, during a system update, the data exchange protocols may not work properly and customer information could get duplicated or fragmented. Ergo, dark data. Not to mention, when Sales and Marketing generate reports based on this faulty data, there are likely to be discrepancies resulting in finger-pointing.
3. Neglected campaign data
As part of campaign efforts, analysts and marketing execs collect and analyze data to formulate strategies. All too often, this data is not put to use ever again. It collects dust while another department duplicates the effort, resulting in a loss of time and a failure of organizational learning efforts.
4. Lack of transparency in sharing data needs
When business processes evolve or change, data needs may also shift. If these alterations aren’t communicated effectively to the data solution team, new types of data might be generated that don't align with the existing data structures or collection methods.
If these changes are not communicated as they happen, the data team is always having to play catch-up. For instance, if a sales team starts recording customer preferences in a new format without informing the data team, it leads to untracked and unutilized data.
5. Lack of executive sponsorship
Any data initiative needs those at the helm to be championing it. If leadership isn’t able to communicate the positive business impact of cohesive, planned data systems, chances are there will be abandoned data sets and poor process hygiene.
Let sleeping data lie - or not?
So far, I have talked about why data turns dark. But why should you care? Even if you have a ton of dark data, why not just let it be?

Dark data is your diamond in the rough, and examining it opens up a treasure trove of potential insights. During my time at Freshworks, we conducted an exercise to shine a light on the dark data lying in reams of support tickets, chat logs and related conversations with customers. This exercise unearthed a number of actionable insights.
- Product development: We recognized that the Admin panel needed revamping. This was not a particularly exciting feature to work on and the product team had not prioritized this until our data showed how much of a pain point it was for customers.
- Support processes: Analyzing the support tickets and customer conversations, we discovered that there were regional variations in customer preference for support channels. Some regions preferred email first, others preferred chat/calls. This helped our customer support team to rejig their processes and cater to customer needs better.
- Marketing efficacy: By comparing the timestamp of conversation IDs with our marketing campaign data, we were able to improve our marketing attribution and link sales outcomes to marketing efforts much more accurately.
All these insights came from looking at just one source of dark data—have I convinced you yet of its hidden power? Well, there’s more.
Looking at dark data can also help with compliance and risk management. For instance, if you were to correlate customer complaints from unutilized feedback forms (i.e, dark data) with product returns in structured databases, you could potentially identify quality control or compliance issues.
A more exciting benefit is that it can make your AI systems better. Dark data often contains unexplored information—unstructured text like customer feedback, call transcripts, etc—that can enrich training datasets for your AI models. With dark data as an ally, AI can also give you more up-to-date insights. It can adapt to current market conditions and customer sentiments quicker when it is able to learn from unstructured dark data like news feeds or social media trends.
The dark side of dark data
If the potential of dark data hasn’t convinced you, think about this. Left unchecked, dark data can be a very costly liability.
The more data you have, the more you need to pay in infrastructure costs. What’s more, with regulations such as GDPR and other privacy laws, ungoverned dark data can be a legal or financial liability that can lead to heavy penalties.
If such a large proportion of your data is unstructured or hidden, is it really secure? It would be an easy task for a perpetrator to misuse sensitive business information that’s laying neglected in the digital nook and crannies of your organization.
Thus, examining your data and purging what isn’t needed isn’t just valuable, it is the sensible thing to do to reduce costs and complexities.
How to illuminate dark data
Okay, say all the key stakeholders are convinced that your organizational dark data needs to see the light. How do you go about it?
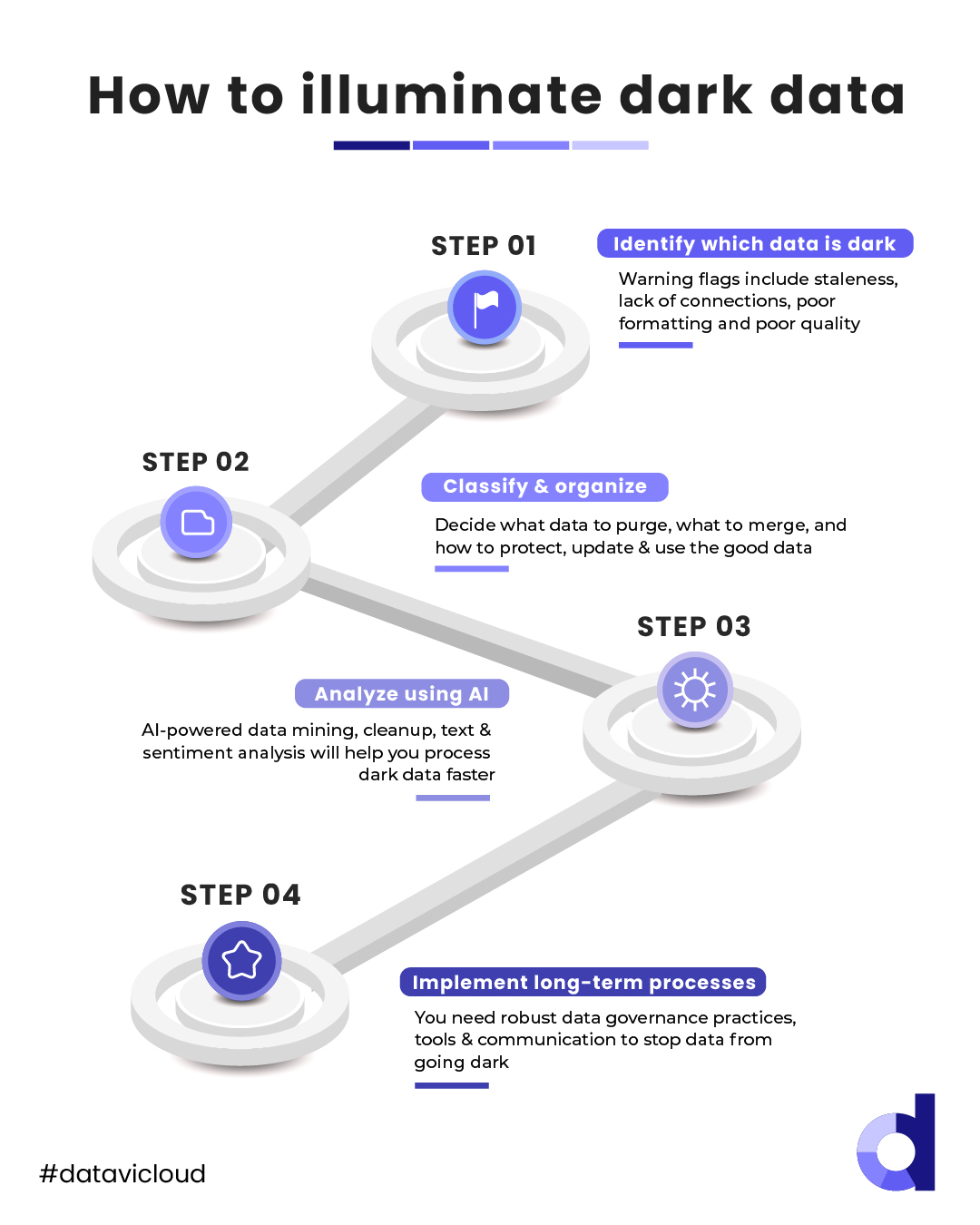
1. Start by identifying dark data
There are certain warning flags that make it likely that a certain data set is dark.
- Staleness: if the data has not been modified in a considerable period of time. The definition of this time period depends on your organization.
- Connections: if there are no data pipelines writing to it or reading from it, chances are that it might be a data silo.
- Quality: poor data quality, for instance, null or duplicate values, is another indicator that the data is dark.
- Formatting: if the dataset is unclassified, unlabeled or untagged, it is a prime candidate for turning dark.
2. Classify and organize the data
Set about organizing the dark data you have identified, by classifying them based on type of information, sensitivity, storage location, format etc. Decide, after thorough consideration, which data sets can be purged. Use ML techniques such as Data Similarity Discovery to get rid of redundant data. Once the dead data is purged, develop a plan for managing the uncovered good data—where you will store it, how you will protect it, how it can be updated, how you will ensure authorization and access rights, and so on.
3. Analyze and extract insights using AI
AI is your foremost ally in all dealings with dark data. It can mine your data to add tags, structure, meta-information etc. It can make sense of hitherto unused information. For example, unstructured data in the form of chatbot interactions and survey related feedback often gets missed in conventional data analytics. But using AI-powered text analytics and sentiment analysis can help you extract valuable pieces of information from these sources. Even if you have only a small data team or even a single engineer, you can still carry out such exercises, thanks to the massive leaps that language models have made.
4. Implement long-term process changes
Once you’ve completed spring cleaning your dark data, you’ll need to put processes in place to ensure that it continues to be properly managed and analyzed.
- Ensure that there is effective communication from the executive team about the importance of managing dark data.
- Plan regular audits of your dark data and training for all stakeholders on how to handle it.
- Invest in data discovery, classification, and security tools, to keep your dark data secure and use it in fresh ways.
The bottomline: Dark data isn’t going anywhere
Data is getting generated at every touchpoint every second of our existence. And storage is not ridiculously expensive (until the data burgeons beyond a tipping point). Therefore, organizations will continue to store much more data than they will ever use.
In attempting to illuminate dark data, there have to be short term solutions, while candidly admitting the flaws in the methodology and trade-off between speed and accuracy. These help break the impasse in decision making and will be valued in fast-paced environments. However, these are no replacements for durable solutions, which can be developed sometimes over a few quarters and in some rare cases over a few years (of course with clear milestones).
Long term solutions will require goal alignment among contributing and affected functions for getting resource-level commitments; perhaps a whole new set of tools, systems, and telemetry; and significant shifts in user behavior and sensitisation about the context. With the right mindset, tools, and robust data governance processes, you can keep a check on dark data and even better, tap into it to unearth a goldmine of insights.
Have you ever taken steps to tame your organization’s dark data? What worked for you?